Simion De
PhD, Biostatistics
Advisor:
Jared Huling
Keywords:
Random-Forest, Covariate-Balancing
Abstract
A critical task in accurately estimating causal effects from observational data is to account for confounding, often achieved by weighting techniques aimed at balancing the distribution of confounders between the treated and control groups. Weighting techniques can be classified in two ways. The first way is based on whether estimating weight is parametric or non-parametric. The second way is based on whether one 1) models the propensity score and inverts it or 2) directly constructs weights that attempt to achieve distributional balance between the treated and the control groups. Parametric methods, both modeling and direct balancing, suffer from model misspecification while balancing techniques suffer from the curse of dimensionality. Methods have been developed to break the curse of dimensionality by identifying confounders among many candidate variables. But these are parametric and focus on modeling the propensity score and therefore subject to bias. In this paper, we propose a nonparametric direct balancing approach that uses a random forest to data-adaptively balance on confounders. Our method uses random forests to jointly model the outcome and treatment based on the covariates. To construct a measure of distributional balance that emphasizes covariates that impact both treatment and outcome, we propose a distance based on the proportion of trees in which two observations appear in the same leaf node, resulting in a distance, sensitive to confounders that can reduce dimensionality while focusing directly on the source of bias in estimating a causal effect. We demonstrate the highly competitive performance of our method using extensive simulations.
Support
This study was supported by NHLBI T32 Cardiovascular Epidemiology and Prevention Pre-doctoral Fellowship
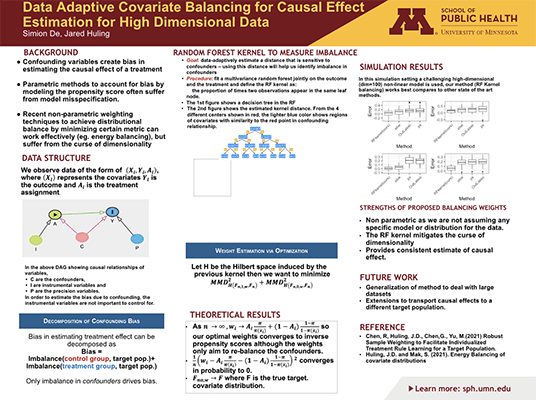
View Poster (PDF)