Many diseases are complex conditions that present themselves in various ways and affect multiple organs in the body. For example, COVID-19 is an illness that ranges in severity based on the strain of the infecting virus and an individual’s own personal health and body characteristics. The onset and course of such diseases can depend on the interplay between several factors, including molecular and environmental conditions within or outside the body. Sorting out which factors are most important and how they work together is a daunting task that requires new approaches.
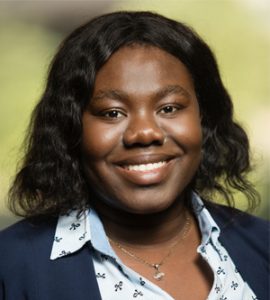
“The traditional approach to investigating the pathology of illnesses focuses on studying a small number of molecules in the body or a single type of data, which cannot fully address the complexity and variation in illnesses,” said Assistant Professor Sandra Safo, an expert in developing statistical methods and computational tools to help identify risk factors for complex diseases. “Statistical and machine learning methods that integrate data from multiple sources — such as molecular, clinical, or demographic sources — will allow us to better understand diseases that are complex and have multiple causes, such as cancer.”
To advance research on integrating data from multiple sources — including molecular, epidemiological, and demographic — Safo has secured a $1.1 million, five-year grant from the National Institutes of Health (NIH) to develop and validate a suite of novel statistical and machine learning methods and software for combining data from multiple sources. The tools will have the potential to identify molecular targets, or “biomarkers,” of a disease, which when explored further, can help explain more about how the disease develops. A biomarker of disease is a molecule found in the body or blood only when a disease or condition is present.
“Our software will generate graphics to characterize data from multiple sources and also generate molecular targets that could be biomarkers and explored further,” said Safo.
The tools will also be useful for identifying subgroups of patients with different characteristics, requiring different therapeutic approaches, and identifying molecular signatures discriminating between different states of disease.
Safo and her team plan to rigorously test the tools with computer simulations, and then use them with publicly available datasets and cohorts to ensure they have “real-world” applications and can significantly contribute to research.
PhD students will also participate in the project to provide them with experiences conducting methodological research, from methods development through testing to findings dissemination.
When the tools are ready, the team will present them in scientific journals and at conferences, disseminate the code/software on GitHub and www.sandraesafo.com, and develop accompanying online tools.